Opinion | Machine learning systems, fair or biased, reflect our moral standards
- While machine learning systems and algorithms are theoretically more objective than humans, this does not necessarily result in different or fairer results
- There needs to be a concerted effort by regulators, governments and practitioners to ensure the technology stems inequities rather than perpetuates them
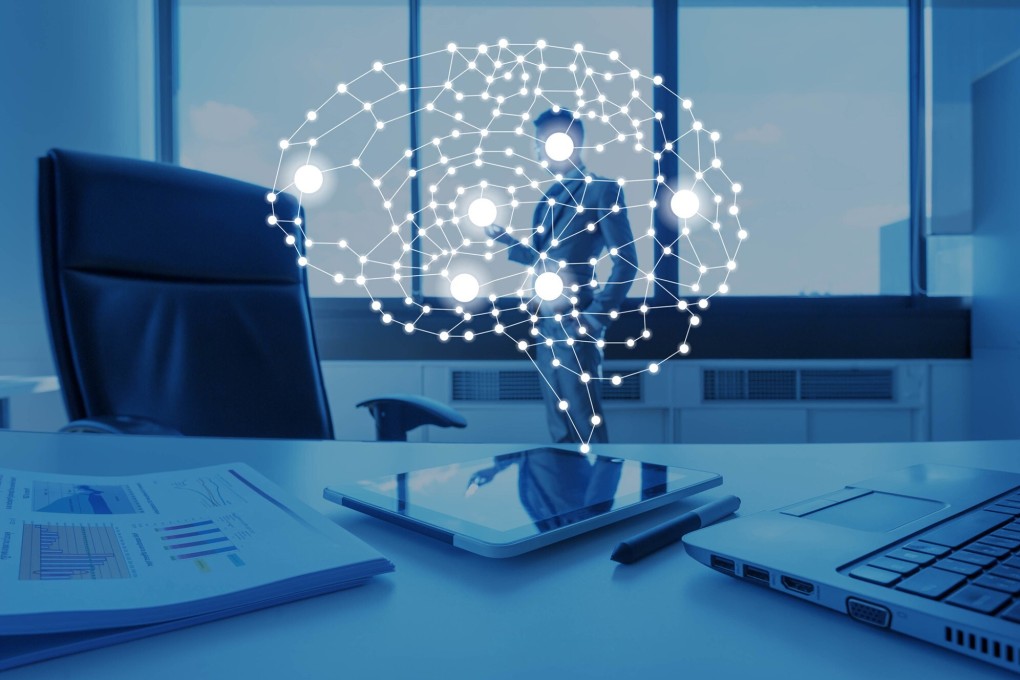
Discrimination in the real world is an ugly reality we have yet to change despite many years of effort. And recent events have made it clear that we have a long, long way to go.
Some companies and governments have turned to automation and algorithms in a bid to remove the human bias that leads to discrimination. However, while machine learning (ML) systems and algorithms are theoretically more objective than humans, the ability to apply the same decision structure unwaveringly does not necessarily result in different or fairer results.
Why is that? Given humans are their progenitors, bias is often built into these ML systems, leading to the same discrimination they were created to avoid. Recently, concerns have emerged that stem from the growing use of these ML systems, when unfiltered for bias, in such areas that could impact human rights and financial inclusion.
Today, ML algorithms are used in hiring, which could be biased by historical pay divides by gender; in parole profiling systems, impacted by historical trends of racial or geography-linked crime rates; and credit decisions, influenced by the economic status of a consumer segment that may have a racial tilt.
These biases stem from flaws in current processes which in turn, colour the training or modelling data set. This can then result in discrimination, and with the growing use of these systems at scale, existing social biases can be further amplified.
How then can we filter out built-in biases and discrimination within these ML systems and make them “fairer” across every segment of society?
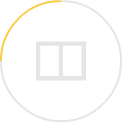